Denoising Diffusion Probabilistic Models
ノイズを除去するステップを何度も繰り返して元画像を復元するやつ
2020
>We present high quality image synthesis results using diffusion probabilistic models, a class of latent variable models inspired by considerations from nonequilibrium thermodynamics.
非平衡統計力学の考察に触発された潜在変数モデルのクラスである拡散確率モデルを用いた高品質な画像合成の結果を発表する
我々の最良の結果は、拡散確率モデルとランジュバン動力学によるノイズ除去のスコアマッチングとの間の新く提案した接続に従って設計された重み付き変分境界で学習することによって得られ、
我々のモデルは自己回帰復号の一般化として解釈できる漸進的損失伸長方式を自然に認める。
>On the unconditional CIFAR10 dataset, we obtain an Inception score of 9.46 and a state-of-the-art FID score of 3.17.
> On 256x256 LSUN, we obtain sample quality similar to ProgressiveGAN. Our implementation is available at this https URL
無条件CIFAR10データセットにおいて、Inceptionスコア9.46、FIDスコア3.17を得ることができました。
256x256 LSUNでは、ProgressiveGANと同程度のサンプル品質が得られている
解説
素人向けの解説
実装レベルの解説
結局ちゃんと理解できるのは実装
>この論文、いきなり読み始めると、大量の数式と難しい概念が出てきて挫折してしまう可能性が高いため、拡散モデルの概要を他の論文やチュートリアル等でおおよそ理解してから挑戦することをオススメします。
元画像にノイズをT回のせる(foward process/diffusion process)
その画像を復元する(reverse process)
復元した画像と予測の差分を損失関数とする
> 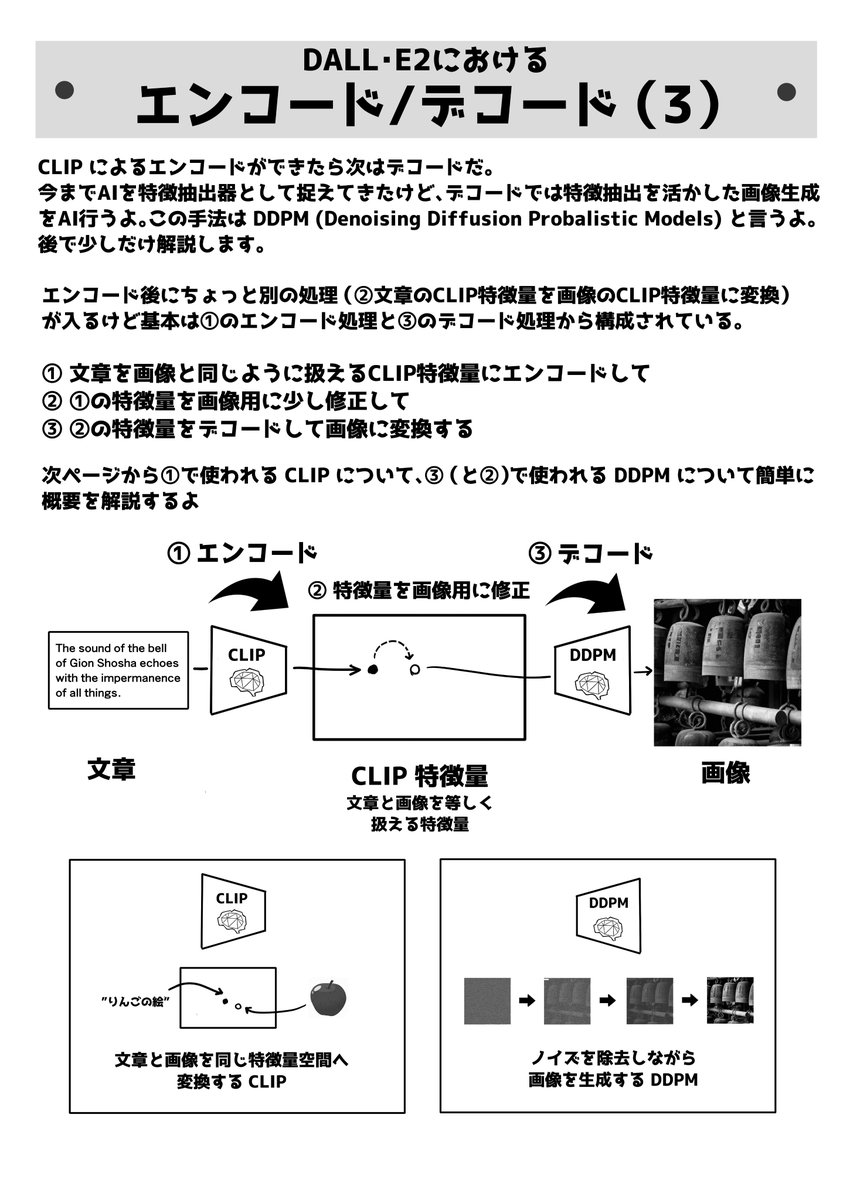
> 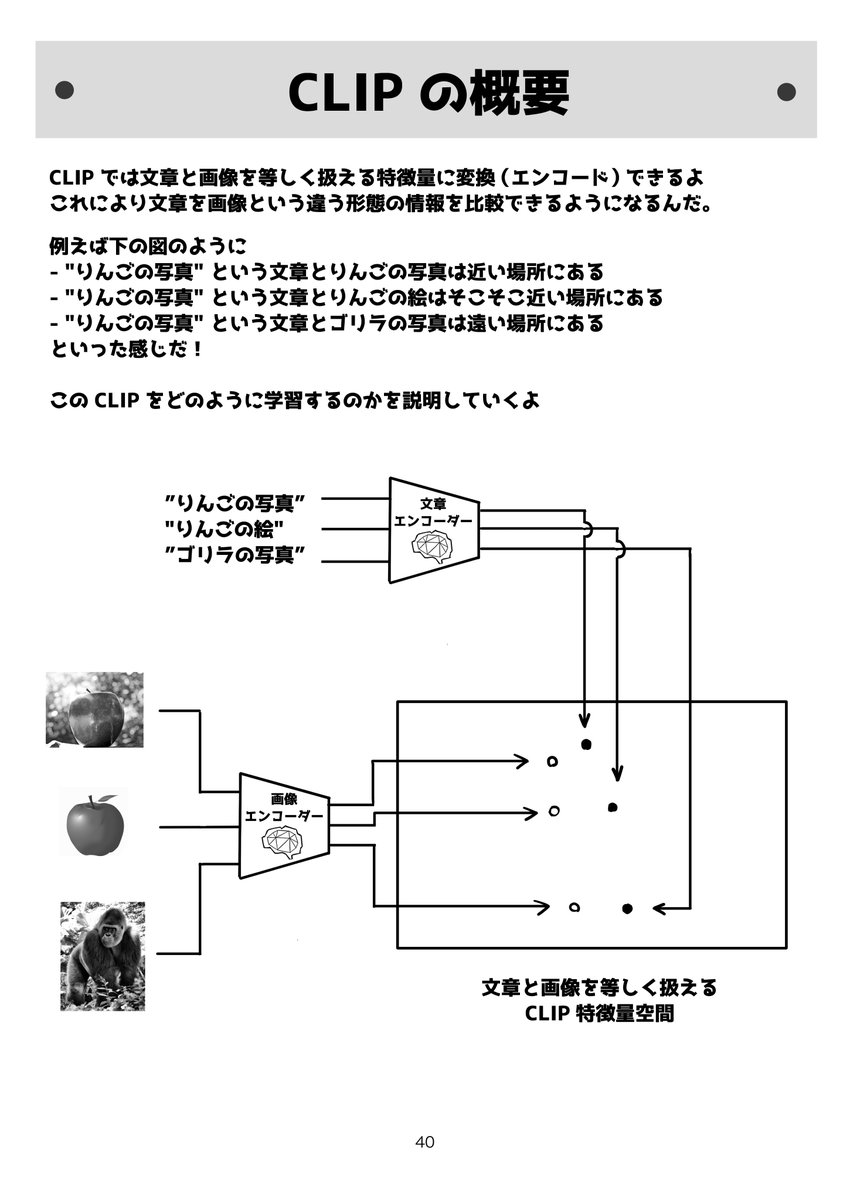
>
/
> 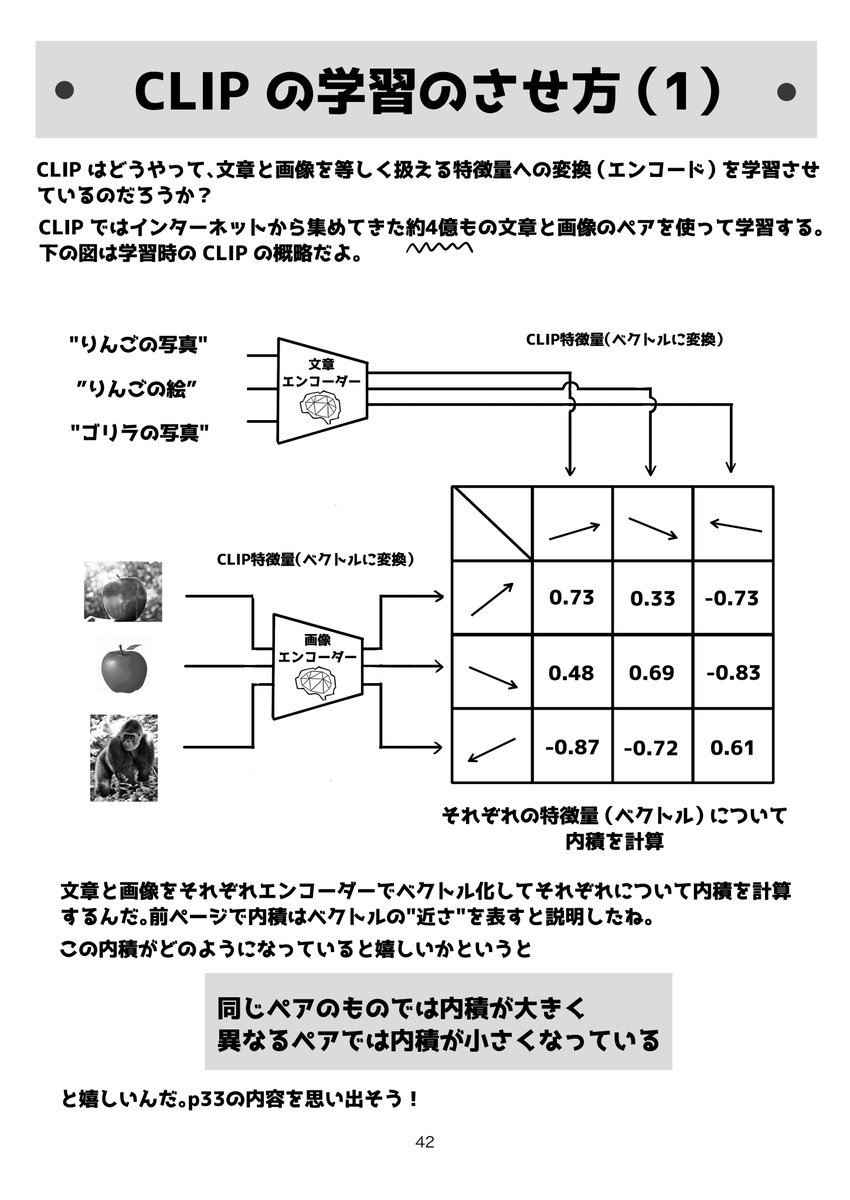
> 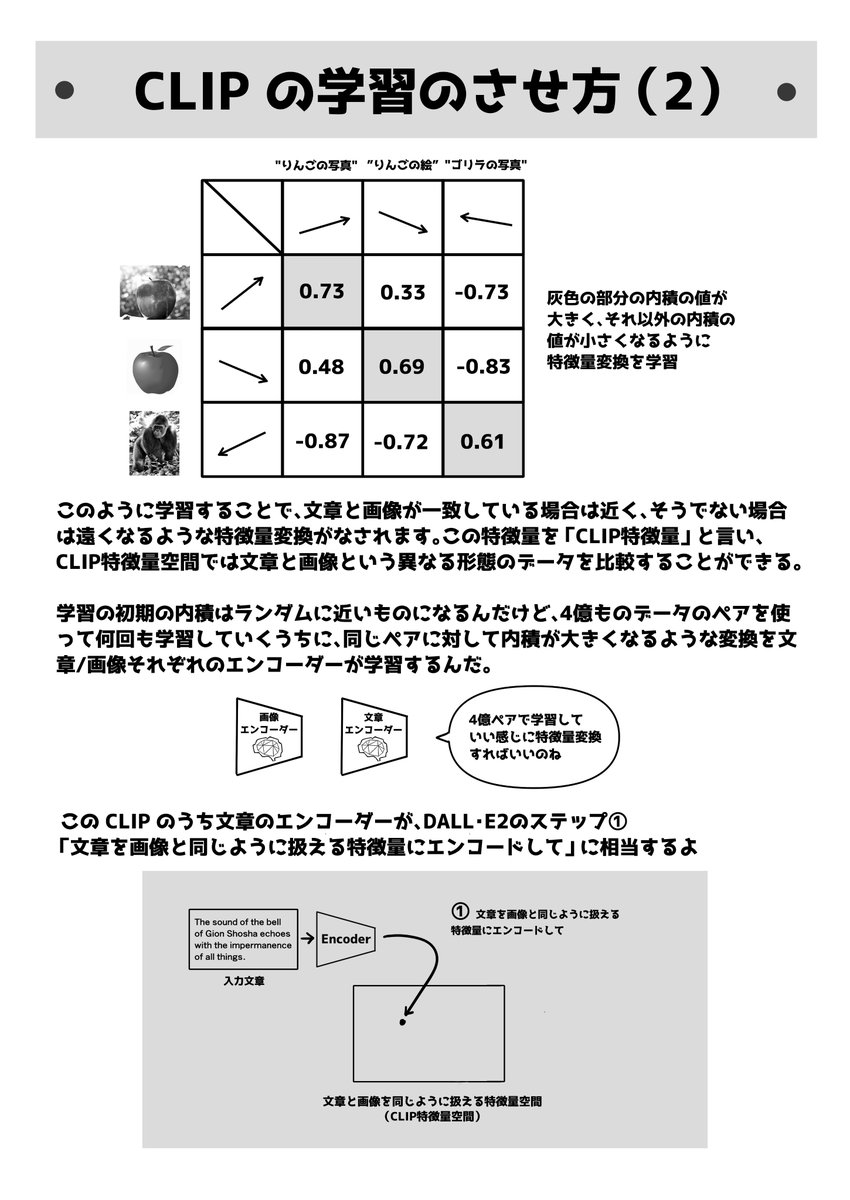
> 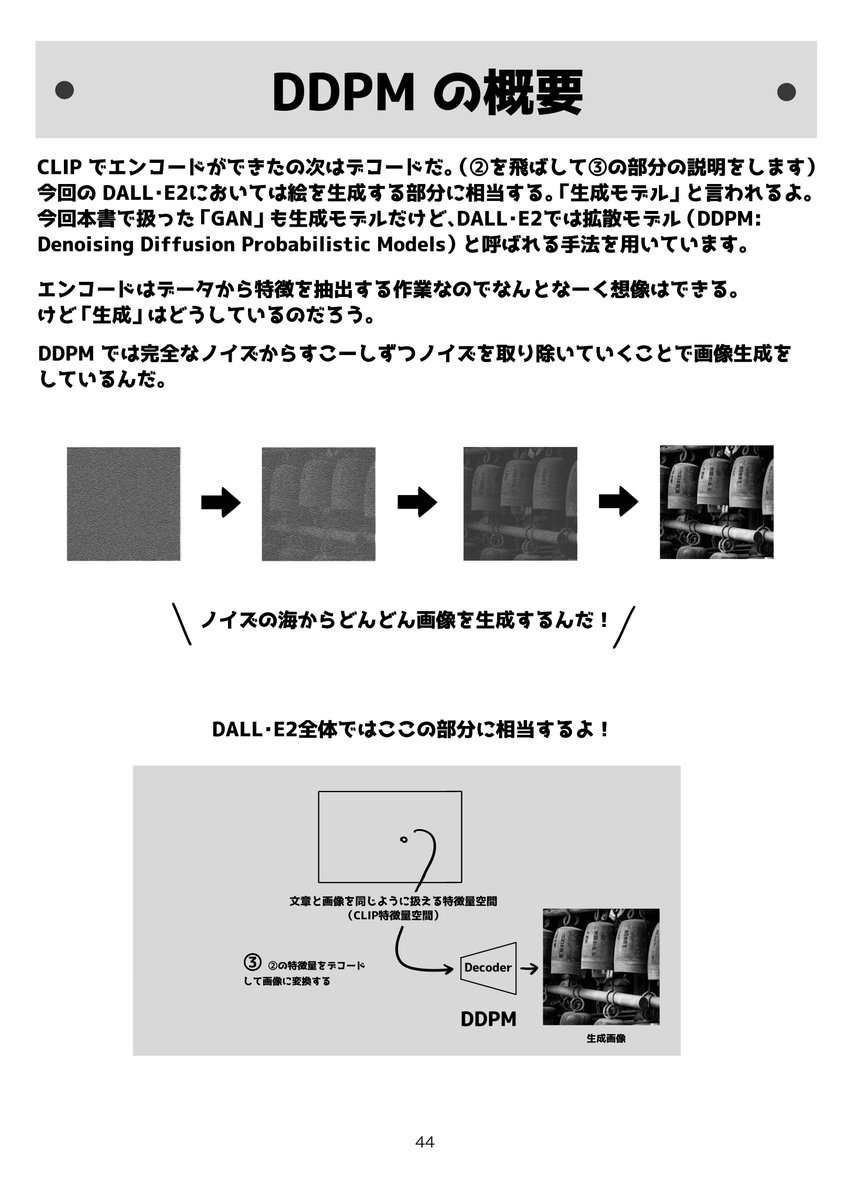
> 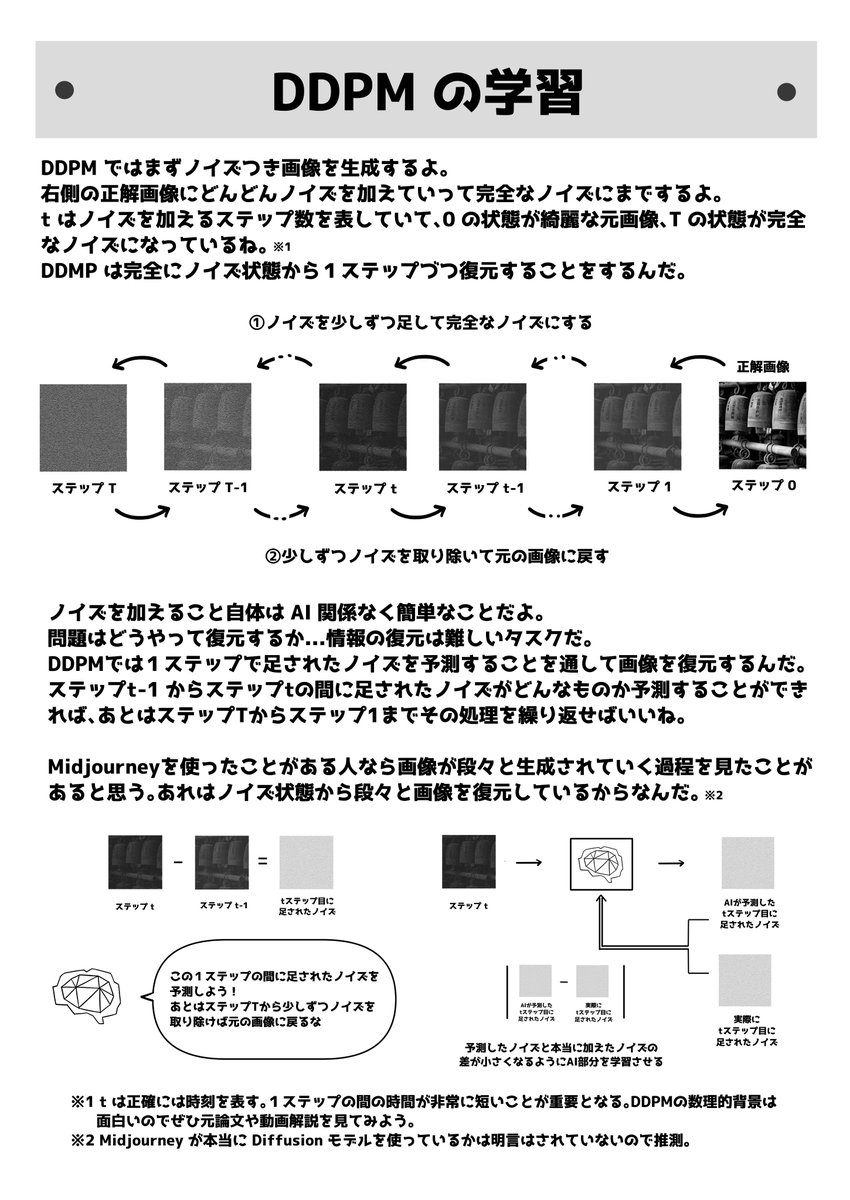
> 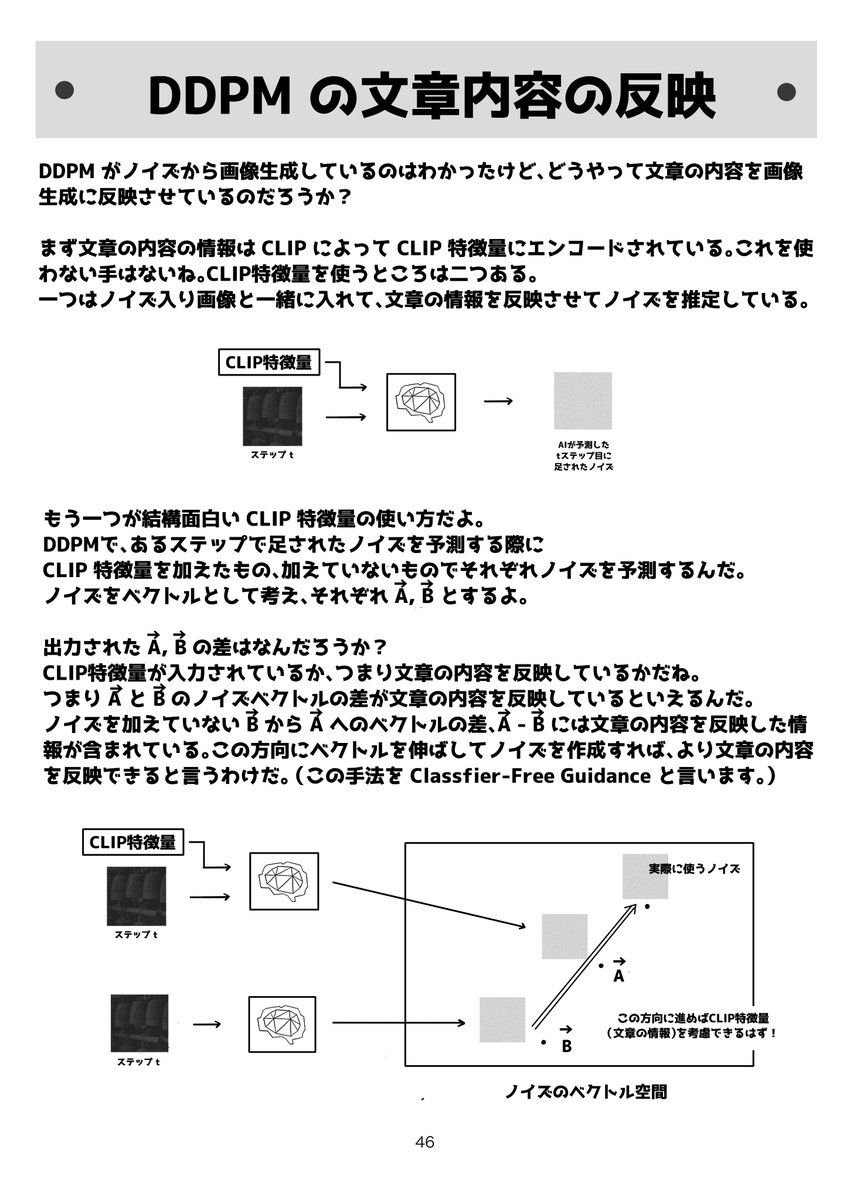
> 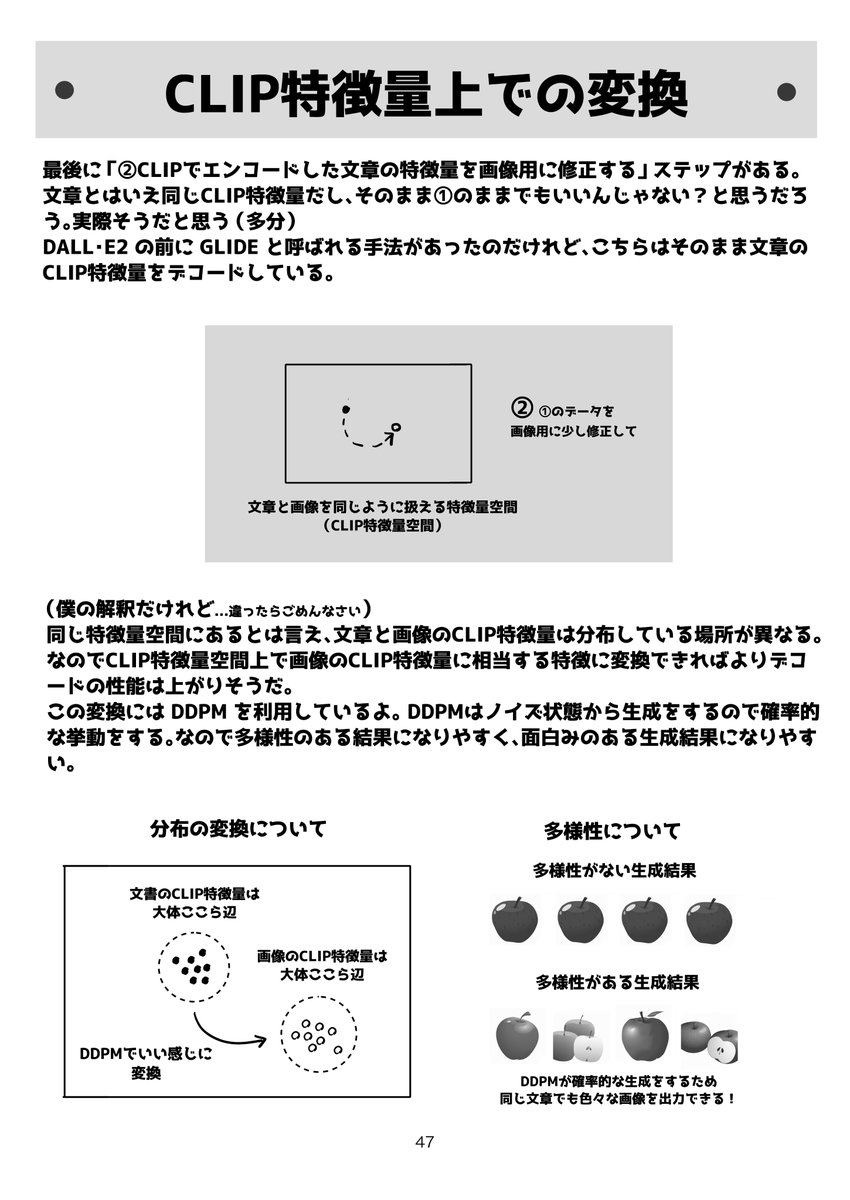
> 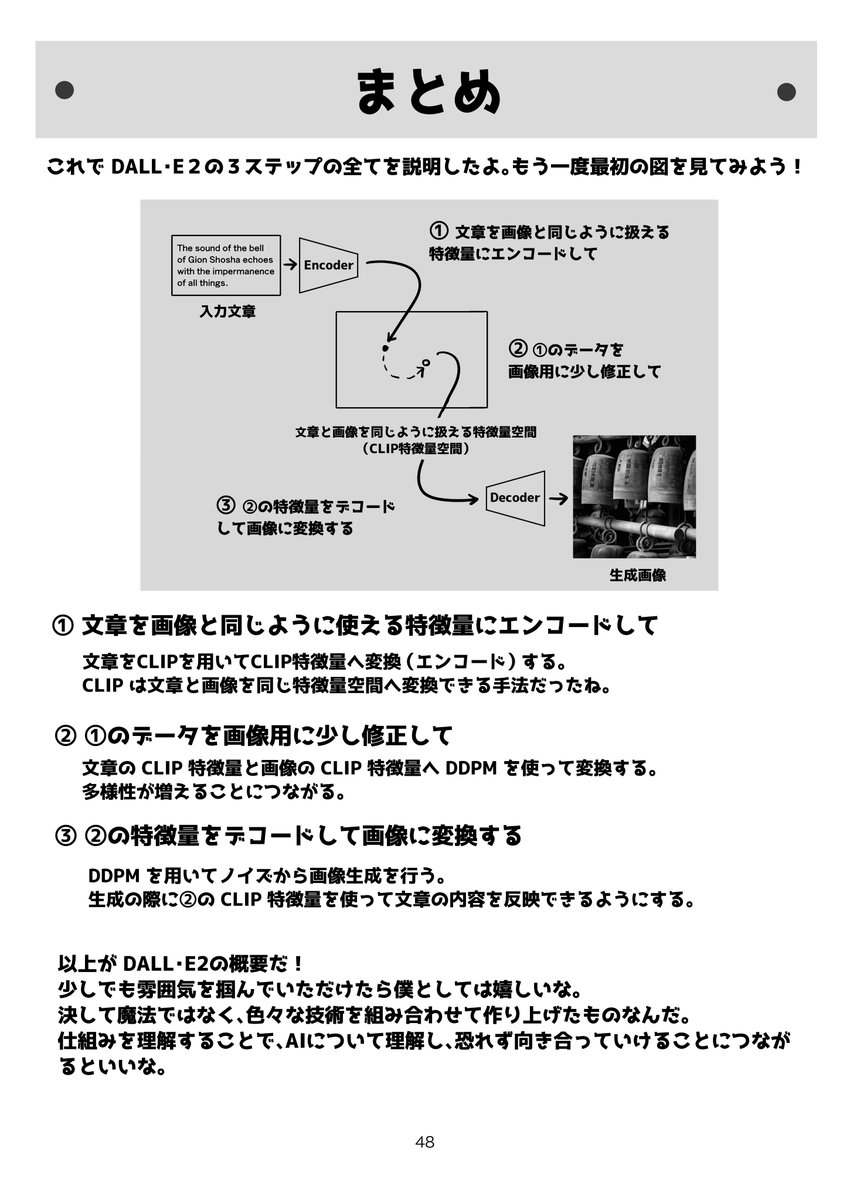
>
/